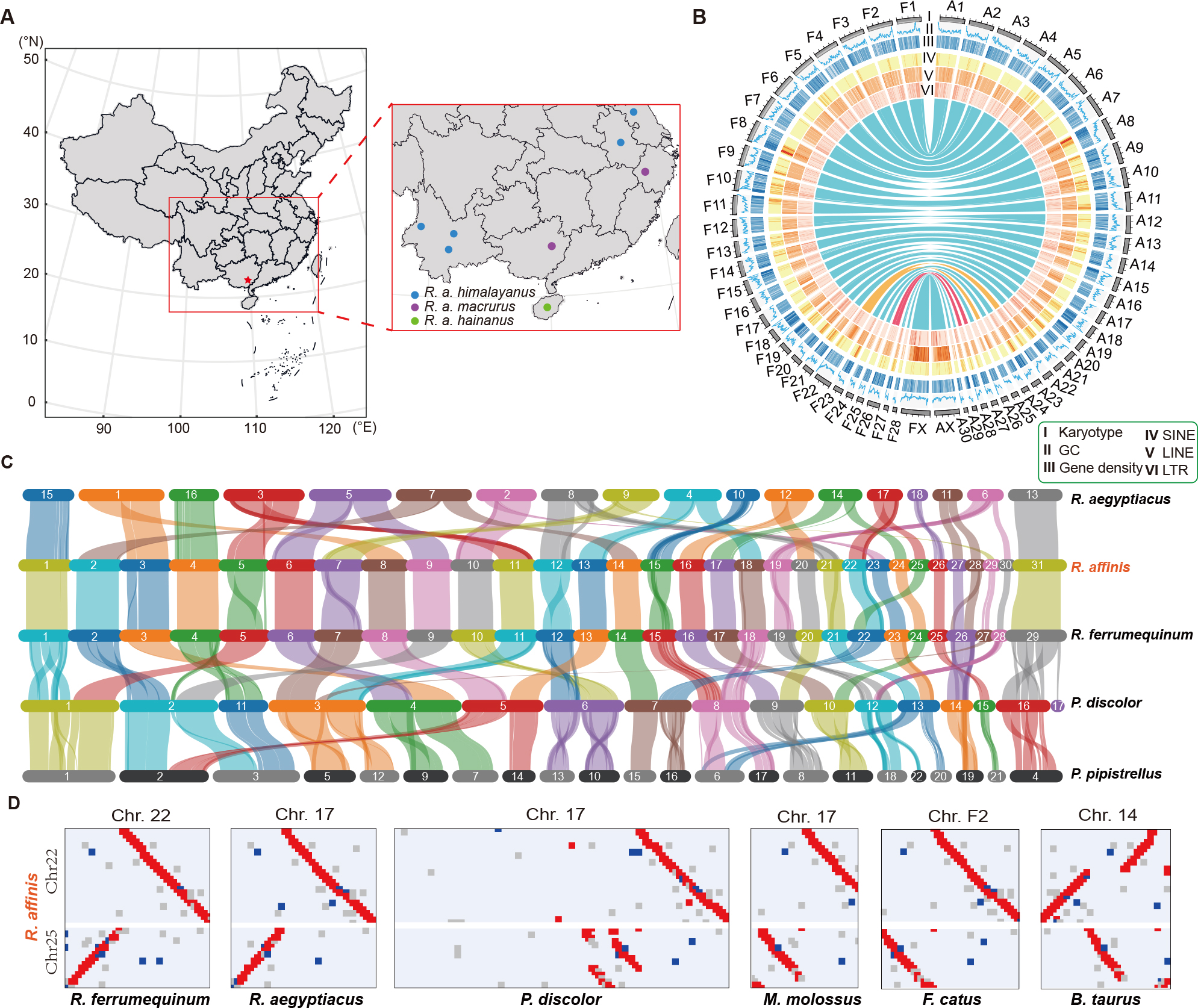
Citation: | Le Zhao, Jiaqing Yuan, Guiqiang Wang, Haohao Jing, Chen Huang, Lulu Xu, Xiao Xu, Ting Sun, Wu Chen, Xiuguang Mao, Gang Li. 2024. Chromosome-level genome and population genomics of the intermediate horseshoe bat (Rhinolophus affinis) reveal the molecular basis of virus tolerance in Rhinolophus and echolocation call frequency variation. Zoological Research, 45(5): 1147-1160. DOI: 10.24272/j.issn.2095-8137.2024.027 |
Horseshoe bats (genus Rhinolophus, family Rhinolophidae) represent an important group within chiropteran phylogeny due to their distinctive traits, including constant high-frequency echolocation, rapid karyotype evolution, and unique immune system. Advances in evolutionary biology, supported by high-quality reference genomes and comprehensive whole-genome data, have significantly enhanced our understanding of species origins, speciation mechanisms, adaptive evolutionary processes, and phenotypic diversity. However, genomic research and understanding of the evolutionary patterns of Rhinolophus are severely constrained by limited data, with only a single published genome of R. ferrumequinum currently available. In this study, we constructed a high-quality chromosome-level reference genome for the intermediate horseshoe bat (R. affinis). Comparative genomic analyses revealed potential genetic characteristics associated with virus tolerance in Rhinolophidae. Notably, we observed expansions in several immune-related gene families and identified various genes functionally associated with the SARS-CoV-2 signaling pathway, DNA repair, and apoptosis, which displayed signs of rapid evolution. In addition, we observed an expansion of the major histocompatibility complex class II (MHC-II) region and a higher copy number of the HLA-DQB2 gene in horseshoe bats compared to other chiropteran species. Based on whole-genome resequencing and population genomic analyses, we identified multiple candidate loci (e.g., GLI3) associated with variations in echolocation call frequency across R. affinis subspecies. This research not only expands our understanding of the genetic characteristics of the Rhinolophus genus but also establishes a valuable foundation for future research.
The genus Rhinolophus, commonly known as horseshoe bats and representing the sole extant genus in the family Rhinolophidae, ranks as the second-largest genus within Chiroptera, comprising 112 species (Simmons & Cirranello, 2024). This genus is distinguished by a suite of unique characteristics that have contributed to its rapid diversification (Csorba et al., 2019). Among these, Rhinolophus displays considerable karyotypic diversity, with diploid chromosome numbers (2n) ranging from 28 to 62 (Mao et al., 2007; Sotero-Caio et al., 2017; Zima et al., 1992). The significant variation in karyotypes suggests that chromosomal fusion and fission events may have played a critical role in driving the rapid speciation observed within this group (Augustijnen et al., 2024; Yoshida et al., 2023). Investigating chromosomal evolution in species with diverse karyotypes, especially those with extreme diploid numbers (e.g., R. affinis with 62) (Zima et al., 1992), could offer valuable insights into the mechanisms underlying such diversification. Additionally, Rhinolophidae is one of the three bat families harboring the highest diversity of viruses, with approximately 80% of known bat viruses identified within this group (Tian et al., 2022). The ability of horseshoe bats to host a broad range of viruses, including being the first species (R. affinis) identified as a reservoir for SARS-CoV-2 (Zhou et al., 2020), suggests the evolution of specialized immune systems that enable these bats to tolerate viral infections. Furthermore, horseshoe bats, along with their sister family Hipposideridae, are unique among Old World bats for their ability to produce constant high-frequency echolocation calls, coupled with Doppler shift compensation (Li et al., 2008; Liu et al., 2012; Teeling et al., 2016; Zhang et al., 2009). This sophisticated echolocation system in the bat world facilitates precision in prey detection and navigation (Jones & Teeling, 2006; Schnitzler et al., 2003).
Recent advancements in comparative genomics based on chromosome-level genome assemblies have provided significant insights into the molecular basis of adaptive traits in various mammalian taxa (Jebb et al., 2020; Shao et al., 2023; Tian et al., 2023). However, genomic data for horseshoe bats remain limited, with only a single high-quality genome assembly available for R. ferrumequinum (Jebb et al., 2020). The primary objective of this study was to construct a high-quality chromosome-level genome for the intermediate horseshoe bat (R. affinis) using a combination of Illumina short-read, Nanopore long-read and Hi-C sequencing technologies. The inclusion of the R. affinis genome will not only facilitate a deeper analysis of the origin and evolutionary history of the Rhinolophidae family but also expand our understanding of the genetic characteristics of ancestral horseshoe bats, as well as specific genetic changes that have occurred during the evolution of this genus.
Rhinolophus affinis, which presents an excellent model for studying the evolution of echolocation, is widely distributed across East and Southeast Asia (Simmons & Cirranello, 2024). In China, R. affinis is represented by three subspecies, including two mainland subspecies (R. a. himalayanus and R. a. macrurus) and one island subspecies (R. a. hainanus), all of which diverged from a common ancestor less than 0.8 million years ago (Ma) (Mao & Rossiter, 2020; Mao et al., 2010). Phylogeographic studies based on mitochondrial and nuclear markers have revealed that R. a. himalayanus has a broad distribution range across mainland China, with a hybrid zone existing between R. a. himalayanus and R. a. macrurus in eastern regions such as Jiangsu and Anhui (Mao & Rossiter, 2020; Mao et al., 2010). Despite the absence of distinguishable morphological characters, these subspecies exhibit distinct echolocation call frequencies, with R. a. himalayanus emitting markedly higher frequencies (87.12±2.04 kHz) compared to R. a. macrurus (73.68±0.74 kHz) and R. a. hainanus (70.85±0.94 kHz) (Mao et al., 2013; Sun et al., 2013; Xie et al., 2017). This suggests rapid adaptation and fixation of these phenotypic traits, potentially driven by population divergence (Kingston & Rossiter, 2004). Currently, the genome-wide signals associated with variations in echolocation call frequency in Rhinolophus are poorly understood. Rhinolophus affinis can serve as an excellent model for investigating the molecular mechanisms underlying echolocation call frequency variation in bats. Further genomic exploration of the genes associated with these changes holds significant potential for elucidating the genetic factors driving the evolution of echolocation, while also providing a deeper understanding of the functional roles of these genes in shaping the observed variations in echolocation frequency among different bat populations.
To determine the genetic mechanisms underlying virus defense in horseshoe bats and to investigate the genetic differences in echolocation among the subspecies of R. affinis, we constructed a chromosome-level genome assembly for R. affinis and performed whole-genome sequencing of multiple individuals for comparative genomic and population genomic analyses. These genomic resources will serve as critical tools for advancing comparative and population genomics research in bats, offering insights into the evolutionary processes shaping these key traits.
For genome sequencing, a single adult male R. affinis was captured by mist net in a cave in Yunnan, China (N25°03'16.7", E103°22'52.5"). Following euthanization by cervical dislocation, fresh tissue (muscle, heart, brain, liver, kidney, cochlea, and gut) was promptly flash-frozen in liquid nitrogen and transferred to a −80°C freezer. For sequencing, we sampled 21 R. affinis individuals from three subspecies (12 R. a. himalayanus, four R. a. macrurus, five R. a. hainanus, Figure 1A; Supplementary Table S1) using a dermatological punch to take 3-mm wing membrane biopsies for each bat. These tissue samples were stored in 95% ethanol at −20°C until DNA extraction. Genomic DNA was extracted using DNeasy kits (Qiagen, Germany) and quantified with a Qubit 2.0 Fluorimeter (Thermo Fisher Scientific, USA). All sampling and tissue collection procedures were approved by the National Animal Research Authority at East China Normal University (approval ID bf20190301).
High-quality genomic DNA was extracted from muscle tissue using a DNeasy Blood and Tissue Kit DNA kit (Qiagen, Germany). Three sequencing technologies were applied to generate a high-quality reference genome. First, an Illumina short-read library with an insert size of 350 bp was constructed and sequenced using the Illumina NovaSeq 6000 platform (paired-end 150 bp, USA). Second, a Nanopore long-read library with >20 kb DNA fragments was created and sequenced using a Nanopore PromethION sequencer (UK). Third, a Hi-C library was generated following previously established procedures (Belton et al., 2012) and sequenced on the Illumina Hiseq platform (paired-end 150 bp, USA). All raw Illumina short reads underwent filtering using fastp v.0.20.1 (Chen et al., 2018), which involved removing sequencing adapters, unpaired, low-quality, and duplicated reads.
The genome size of R. affinis was estimated based on Illumina-filtered reads using Jellyfish v.2.3.0 (Marçais & Kingsford, 2011) by constructing a k-mer count histogram with 19, 21, 23, and 25 mers. Heterozygosity and the rate of duplication were estimated using GenomeScope v.2.0 (Ranallo-Benavidez et al., 2020). The filtered Nanopore reads were assembled using FLYE v2.8.1-b1676 (Kolmogorov et al., 2019) with parameters “--nano-raw --iterations 2”. Illumina short reads were then employed to polish the assembled contigs using NextPolish v.1.3.1 (Hu et al., 2020) with default parameters. Duplicated sequences were identified and removed with PURGE_DUPS v.1.2.5 (Guan et al., 2020). Juicer v.1.5.7 (Durand et al., 2016b) and 3D-DNA v180922 (Dudchenko et al., 2017) were used to construct a chromosome-level assembly based on Hi-C reads. Juicebox Assembly Tools v.1.9.9 (Durand et al., 2016a) was applied for the manual correction of chromosomal structures.
Three methods were used to evaluate genome assembly quality. First, Benchmarking Universal Single-Copy Orthologs (BUSCO) v.4.1.2 was employed to assess genome integrity with the parameters “-m genome -l mammalia_odb10”. Second, synteny analysis was performed between the high-quality genomes of R. affinis and R. ferrumequinum (GCA_004115265.2). Third, the mapping rates of the Illumina short reads and assembled transcriptomes were estimated using Trinity v.2.13.2 (Grabherr et al., 2011) based on RNA sequencing (RNA-seq) data from different tissues to the assembled whole genome of R. affinis.
Repetitive sequences were annotated using both de novo and homology-based predictions. The de novo repeat library was constructed using RepeatModeler v.1.0.11 (http://www.repeatmasker.org/RepeatModeler/). All transposable elements were subsequently identified using RepeatMasker v.4.0.7 (http://repeatmasker.org/) by comparing them against a combined dataset consisting of the Repbase transposable element library and the built de novo repeat library. Tandem repeats and simple sequence repeats (SSRs) were predicted using TRF v.4.09 (https://tandem.bu.edu/trf/trf.html) with default parameters. To explore the evolution of transposable elements in bats, the insertion time of each TE group was calculated using the formula: Insertion time=K/2r, where K is the Kimura value and r is the evolutionary rate, acquired through genome divergence time analysis.
Three approaches were applied for genome annotation, including ab initio prediction, RNA-seq-based prediction, and homology-based prediction. Ab initio prediction was performed using Augustus v.3.3.1 (Stanke et al., 2006), with human gene sets used to train Augustus, GlimmerHMM v.3.0.4 (Majoros et al., 2004), and GeneScan v.1.0 (Aggarwal & Ramaswamy, 2002). For RNA-seq-based prediction, previously published RNA-seq reads from six different tissues (brain, liver, muscle, heart, small intestine, and cochlea, Ding et al., 2021) of R. affinis were aligned to the reference genome using STAR v.2.7.3 (Dobin et al., 2013). Transcriptomes for each tissue were then assembled using Trinity v.2.13.2 (Grabherr et al., 2011), and coding sequences were identified using TransDecoder v.5.5.0 (Haas et al., 2013). Whole protein sequence data of nine mammalian species were downloaded from the NCBI dataset, including six bat species (Rhinolophus ferrumequinum, Rousettus aegyptiacus, Phyllostomus discolor, Myotis myotis, Pipistrellus kuhlii, and Molossus molossus) and three non-bat species (Felis catus, Bos taurus, and Homo sapiens) (Supplementary Table S2). Homology-based gene prediction for R. affinis was performed using the GeMoMa pipeline v.1.6.1 (Keilwagen et al., 2016) with the protein data downloaded for the nine selected mammals as input queries. Finally, EVidenceModeler v.1.1.1 (Haas et al., 2008) was used to combine the results of all predictions based on weighted consensus. For functional annotation, all annotated coding genes of R. affinis were searched against the SwissProt and Nr databases using diamond v.2.0.8.146 (e-value, 1e-3). Gene Ontology (GO) and Kyoto Encyclopedia of Genes and Genomes (KEGG) annotations were performed using eggNOG-Mapper v.1.0 (Cantalapiedra et al., 2021).
OrthoFinder v.2.4.0 (Emms & Kelly, 2015) with default parameters was used to identify one-to-one orthologs across R. affinis, seven additional bat species (R. ferrumequinum, Hipposideros armiger, Rousettus aegyptiacus, Phyllostomus discolor, Myotis myotis, Pipistrellus kuhlii, and Molossus molossus), and two outgroup species (Felis catus and Bos taurus) (Supplementary Table S2). The protein sequences of all orthologous genes were then aligned using prank v.170427, with the alignments further trimmed using trimAl v.1.4.rev22 (Capella-Gutiérrez et al., 2009). A phylogenetic tree was constructed using RAxML v.8.2.12 (Stamatakis, 2014) based on the concatenated protein alignments with the PROTGAMMAJTT protein substitution model, and bootstrap tests were estimated with 100 replicates. Divergence time estimates for the bats and outgroups were inferred using the “r8s” v.1.81 program (Sanderson, 2003). Time constraints (38–56 Ma for the split of Hipposideridae and Rhinolophidae, 38–56 Ma for the common ancestor of Molossidae, Vespertilionidae, and Miniopteridae; 47.8–61.6 Ma of the deepest divergence of Yangochiroptera, and 47.8–66 Ma for the earliest bat ancestor) were used for calibration, as suggested in previous research (Foley et al., 2016).
CAFE v.4.0 (https://github.com/hahnlab/CAFE) was used to analyze gene family expansion and contraction across seven bat species with the parameters “lambda -s -t” (De Bie et al., 2006). Gene families with an estimated P-value lower than 0.05 were considered significantly expanded or contracted. The single-copy orthologous genes for the examined taxa were used to calculate the ratio of nonsynonymous substitutions per nonsynonymous site to synonymous substitutions per synonymous site (Ka/Ks) using the PAML package v.4.9 (Yang, 2007). The branch-site model was applied to evaluate the signatures of positive selection acting on each gene in the foreground branches (R. affinis branch and ancestor of R. affinis/R. ferrumequinum branch, respectively). Significantly positively selected genes (PSGs) were determined using the Chi-Squared test, with “<0.01” indicating very significant and “>0.01 and <0.05” indicating significant. Functional and pathway enrichment analyses of the selected genes were performed using Metascape v.3.5.20230501 (http://metascape.org/), including the GO, KEGG, and WikiPathways databases.
To analyze the variation in genomic structures between the chromosome-level genome assemblies of two Rhinolophus species (R. affinis and R. ferrumequinum) and other bat species, two approaches were applied for genomic collinearity comparison. The first approach involved cross-gene protein sequence blasting between bat species pairs using BLATSP v.2.10.1+ (e-value: 1e-10). The second approach identified whole-genome synteny blocks across the compared bat genomes using ColinearScan v.1.0.1 (Wang et al., 2006). The results of these analyses were summarized and visualized in dot plots using in-house developed PERL scripts.
The whole-genome DNA sequencing library (insert size 300 bp) was built and sequenced using an Illumina HiSeq 4000 sequencer (150 bp paired-end, USA). For each R. affinis sample, we generated approximately 30 Gb of resequencing data (about 15× genome coverage) (Supplementary Table S1). Raw resequencing data were trimmed using Fastp with default parameters. For each sample, the trimmed short reads were mapped to the reference genome of R. affinis using BWA v.0.7.17-r1188 (Li & Durbin, 2010) and SAMtools v.1.8 (Li et al., 2009). Polymerase chain reaction (PCR)-induced duplicate reads were removed with PICARD v.1.56 (http://broadinstitute.github.io/picard/). During SNP calling, the mapped reads were locally realigned using IndelRealigner in the Genome Analysis Toolkit (GATK v.3.8) (McKenna et al., 2010). The HaplotypeCaller function in GATK was used for SNP and INDEL discovery and genotyping. Identified population variants were filtered based on specific criteria: SNP with “DP<161; DP>1453; QD<2.0; FS>60.0; MQ<40.0; MQRankSum<-12.5; ReadPosRankSum<-8.0; SOR>3.0”. SNPs in repeated regions were also removed. A total of 50 626 625 SNPs were retained for all R. affinis individuals. Relatedness among individuals was determined using KING v.2.3.0 (https://www.kingrelatedness.com). Furthermore, PLINK v.1.9 (Purcell et al., 2007) was used to remove SNPs with possible linkage disequilibrium, resulting in 5 188 130 unlinked SNPs for all involved R. affinis individuals.
Phylogenetic analysis was performed based on all SNP data using MEGA v.11 (Tamura et al., 2021), with the neighbor-joining (NJ) tree constructed with 1 000 bootstraps. The IQtree v.1.6.12 program (Nguyen et al., 2015) was used to build the maximum-likelihood (ML) tree with the parameters “iqtree -s ZJ.phy -m MFP -redo -bb 1000 -nt 32”. Principal component analysis was performed to cluster all R. affinis individuals using the smartpca program within the EIGENSTRAT v.2.0 package (Patterson et al., 2006). Genome-wide admixture of R. affinis populations was quantified using ADMIXTURE v.1.3.0 (http://dalexander.github.io/admixture/) for each possible group number (K from 1 to 4).
The pairwise sequentially Markovian coalescent (PSMC) method (Li & Durbin, 2011) was employed to estimate the historical fluctuations in effective population size (Ne) across the three R. affinis subspecies. One individual with the highest sequencing depth from each subspecies population was selected to ensure the quality of the consensus sequence. For the PSMC analysis, parameters were set to -N25, t15, -r5, -p “4+25×2+4+6”, with 100 bootstrap replicates. Furthermore, MSMC2 v2.1.1 (https://github.com/stschiff/msmc2) was applied to infer population history using four haplotypes per subspecies, with the key model set to “1×2+25×1+1×2”. Prior to these analysis, the nucleotide mutation rate (μ) for R. affinis was calculated using the equation: μ=D×g/2T, where D is the observed sequence difference between R. affinis and R. ferrumequinum estimated by mummer4 v.4.0.0rc1 (Kurtz et al., 2004), T is the divergence time of these two species (17.52 Ma), and g is the generation time (2.5 years per generation) (Kumar et al., 2022). The estimated mutation rate for R. affinis was 4.62e-9 mutations per site per generation (Zhao et al., 2021).
Genome-wide heterozygosity of the R. affinis populations was calculated as the proportion of identified heterozygous sites in the R. affinis genome using PLINK v.1.9. Nucleotide diversity was calculated using VCFtools v.0.1.16 with the parameters: --window-pi 50000 --window-pi-step 20000 --maf 0.05 --max-missing 0.90 (Danecek et al., 2011). Linkage disequilibrium patterns of the R. affinis populations were evaluated using PopLDdecay v.3.41 (Zhang et al., 2019). This approach involved a genome-wide scan of selection signals using the genetic differentiation index FST and nucleotide diversity π (Feng et al., 2023; Zhang et al., 2022).
To investigate potential genetic divergence in candidate hearing genes associated with phenotypical variations in echolocation call frequency, the genetic divergence index (FST) and nucleotide diversity (π) were applied to compare R. a. himalayanus and the other two subspecies. Given the similar echolocation call frequencies and close genetic backgrounds of R. a. macrurus and R. a. hainanus, as well as their recent divergence and lack of distinction in admixture analysis, these two populations were combined into a single group for comparison with R. a. himalayanus. Sliding windows (50 kb window size and 20 kb step) were used to calculate genome-wide FST and π ratio values using VCFtools v.0.1.16. Z-transformation was applied to the FST values to obtain ZFST values, and the π ratio was log transformed (loge). The top 5% of windows with the highest ZFST and maximum ln(π ratio) values were identified as candidate outliers under selection in the R. a. himalayanus population. Conversely, the bottom 5% of windows with the lowest ZFST and ln(π ratio) values were identified as candidate selection outliers in the R. a. macrurus/R. a. hainanus group. It is important to note that genetic drift can also potentially influence unique genomic features or regions (Funk et al., 2016). However, given the relatively large Ne for each R. affinis subspecies, the impact of genetic drift is likely to be minimal in this context.
Functional enrichment analysis was performed using Metascape v.3.5.20230501 (http://metascape.org/). Significant GO terms and KEGG pathways were determined with a corrected P-value (Q-value<0.05) using Benjamini-Hochberg multiple test correction. Redundant terms or pathways were reduced using the REVIGO clustering algorithm v.1.8.1 (http://revigo.irb.hr/) with default settings.
A high-quality chromosome-level reference genome of R. affinis was assembled using Illumina short reads (~60 Gb), Nanopore long reads (~167 Gb), and Hi-C reads (~145 Gb) for chromosomal anchoring. The initial assembly resulted in 616 contigs (N50>30 Mb) with a genome size of 2.02 Gb. Remarkably, 99.97% of the contigs were successfully anchored to 32 chromosomes (30 autosomes, X and Y chromosomes) (Table 1; Supplementary Figure S1 and Table S3), consistent with the reported karyotype of R. affinis (Mao et al., 2007). The re-mapping rates of the Illumina short reads and RNA-seq data to the assembled R. affinis genome were 99.27% and 98.19%, respectively. The completeness of the assembled genome was 93.2% based on BUSCO analysis. A total of 21 168 protein-coding genes were annotated, 20 939 (98.92%) of which were also functionally annotated in the GO and KEGG databases (Supplementary Table S4). Comparative genomic analysis between R. affinis and R. ferrumequinum revealed highly conserved collinearity in their genomes (Figure 1B).
Type | G1 (Contig level) | G2 (Contig level) | G3 (Contig level) | Hi-C (Chromosome level) |
Number of contigs/scaffolds | 1 166 | 1 166 | 616 | 32 |
Contig/scaffold N50 (bp) | 30 381 862 | 30 560 113 | 30 201 243 | 92 909 886 |
Contig/scaffold N90 (bp) | 4 454 582 | 4 480 356 | 4 876 479 | 38 872 647 |
Longest contig/scaffold (bp) | 81 976 908 | 82 468 273 | 82 468 273 | 107 778 528 |
Average contig/scaffold length (bp) | 1 771 639 | 1 782 631 | 3 287 950 | 63 275 039 |
Total genome length (bp) | 2 065 732 003 | 2 078 548 679 | 2 025 377 528 | 2 024 801 270* |
*: Unanchored contig base count is not included. |
A total of 573.78 Mb of repetitive elements were identified in R. affinis genome, comprising various types of transposable elements, including DNA (15.36%), LINE (41.88%), LTR (18.67%), SINE (15.53%), and others (8.56%) (Supplementary Table S5). Analysis of historical transposable element insertions revealed that, following the divergence from Pteropodidae, the ancestor of Rhinolophoidea experienced a specific expansion of DNA elements, including TCMAR-MARINER, TCMAR-TC1, and TCMAR-TC2 (Supplementary Figure S2). Notably, a distinct expansion of the ACADEM-1 DNA element was observed in R. affinis (Supplementary Figures S2, S3). In contrast to other Yinchiroptera species, the common insertion of ZISUPTON and PIGGYBAC elements was not detected in R. affinis (Supplementary Figures S2, S3). Furthermore, although R. affinis and R. ferrumequinum shared similar genome sizes and comparable composition and total amounts of repetitive elements, differences were noted in the insertion timing and rate of these elements between the two species (Supplementary Figure S2).
A genome collinearity comparison was conducted between the R. affinis genome and four other high-quality chromosome-level bat genomes (R. ferrumequinum, R. aegyptiacus, P. discolor, and P. pipistrellus, accessed October 2020). The results revealed multiple chromosomal rearrangement events, including fissions, fusions, and translocations, among the five bat genomes (Figure 1C). Compared with the R. affinis genome, 44, 56, 66, and 84 collinear gene blocks were identified in the genomes of R. ferrumequinum, R. aegyptiacus, P. discolor and P. pipistrellus, respectively (Supplementary Figures S4–S6). These findings suggest a correlation between genomic structures and phylogenetic distance.
The collinearity comparison results indicated that chromosome 22 of R. ferrumequinum was homologous to chromosomes 22 and 25 of R. affinis. Further syntenic comparisons among different bats suggested a conserved chromosomal structure in R. ferrumequinum chromosome 14, with chromosomal fission events likely occurring later to form chromosomes 22 and 25 in R. affinis (Figure 1D). Notably, two endogenous retrovirus genes, RVK-6 and HERVK_113, were detected near the breakage point of R. ferrumequinum chromosome 14, which have been previously implicated in mediating chromosomal rearrangements (Hughes & Coffin, 2001; Weckselblatt & Rudd, 2015).
Using OrthoFinder, 18 607 homologous gene families and 11 329 single-copy homologous genes were identified across all bat species studied and the two outgroups (Supplementary Tables S6, S7). A concatenated data matrix of single-copy homologous genes was applied to reconstruct the ML tree (Supplementary Figure S7), yielding topologies consistent with previously published findings (Stoffberg et al., 2010). Divergence time estimation indicated approximately 16.18 Ma between the divergence of R. affinis and R. ferrumequinum, and 38 Ma between Rhinolophidae and Hipposideridae (Figure 2A).
Further analysis identified 196 gene families, comprising 676 genes that expanded during the evolution of R. affinis following its divergence from R. ferrumequinum. Functional enrichment analysis revealed that these expanded genes were significantly associated with multiple immune-related functions, including viral genome integration into host DNA (GO:0044826), viral translational readthrough (GO:0039705), DNA integration (GO:0015074), and positive regulation of double-strand break repair via nonhomologous end joining (GO:2001034) (Figure 2A, B; Supplementary Table S8). For the ancestral Rhinolophidae branch, 49 expanded gene families were identified, showing functional enrichment associated with sensory perception of chemical stimulus (GO:0007606) and regulation of endoribonuclease activity (GO:0060699) (Supplementary Table S9).
Further comparison of multiple genomes highlighted that Rhinolophidae species (R. affinis and R. ferrumequinum) possessed the longest major histocompatibility complex (MHC) regions (1.81 Mb in R. affinis and 1.53 Mb in R. ferrumequinum), and the highest number of genes (27, including 14 MHC-II genes) (Supplementary Table S10; Figure 2C) compared to other bat species. These results suggest an expansion of MHC genes in the common ancestor of the Rhinolophidae lineage. In addition, compared to other bats, Rhinolophidae species contained more gene copies of HLA-DQB2 (three and one (or none) in Rhinolophidae and other bats, respectively), a gene specifically expressed in human epidermal Langerhans cells (Lenormand et al., 2012) and associated with systemic lupus erythematosus (Barcellos et al., 2009), rheumatoid arthritis (Kochi et al., 2004), and hepatitis B (Chang et al., 2014; Xu et al., 2017).
The synonymous and nonsynonymous substitution rates of the 11 329 identified single-copy homologous genes were calculated to test the signatures of natural selection on different Rhinolophoidea branches. In total, 504 significant PSGs were identified on the R. affinis branch after its divergence from R. ferrumequinum (Supplementary Table S11). Functional analysis indicated that these PSGs were significantly enriched in sensory organ development (GO: 0007423), inflammatory response (GO:0006954), and various immune-related items, such as regulation of humoral immune response (GO:0002920), immune effector process (GO:0002252), and complement system (WP2806) (Figure 2D; Supplementary Figure S8 and Tables S12–S14). Similarly, the 649 PSGs detected on the ancestral branch of Rhinolophidae (Figure 2b; Supplementary Table S15) were associated with inflammatory response (GO:0006954), defense response to virus (GO:0051607), and other immune-related functions (Supplementary Tables S16–S18). Many identified PSGs of Rhinolophidae (e.g., CASP8 and BCL2L14) were functionally related to apoptosis, an important mechanism of virus removal (Benedict et al., 2002). Notably, several PSGs were involved in the SARS-CoV-2 signaling pathway, including C1S, CASP8, and CD2, and the well-reported ACE2 gene (Figure 2E; Supplementary Figure S9). Collectively, these findings suggest that the expanded gene families and PSGs in the evolution of the Rhinolophidae clade exhibit significant functional enrichment related to immune processes.
In addition, multiple DNA repair-related genes, such as PRKDC and ATM, were found to be under positive selection on the ancestral branch of Rhinolophidae. These genes play crucial roles in non-homologous end joining (NHEJ) and homologous recombination (HR), which contribute to chromosomal rearrangements. Specifically, the PRKDC gene functions in DNA double-strand break repair and recombination (Lees-Miller & Meek, 2003), while ATM encodes an essential cell cycle checkpoint kinase whose phosphorylation is involved in the cell’s response to DNA damage and genome stability (Bartek et al., 2007; Traven & Heierhorst, 2005) (Figure 2F, G).
The ML and NJ trees were constructed using concatenated population genomic SNP data from the three R. affinis subspecies. The phylogenetic analyses supported an initial split of R. a. himalayanus, followed by the divergence of R. a. macrurus and R. a. hainanus (Figures 1A, 3A; Supplementary Figure S10). This clustering pattern was further corroborated by principal component analysis (PCA) (Figure 3B; Supplementary Figure S11). However, admixture analysis with two clusters (K=2) indicated that R. a. himalayanus and the mixed group of R. a. macrurus and R. a. hainanus best fit the data (Figure 3A; Supplementary Figure S12). These results are consistent with our recent study based on sequence capture data (Mao & Rossiter, 2020).
The PSMC and multiple sequentially Markovian coalescent (MSMC) methods were applied to infer historical changes in Ne for the three R. affinis subspecies. Results revealed that the three subspecies shared a common ancestor 0.9 to 8 Ma (Figure 3C). The inferred split between R. a. himalayanus and the common ancestor of R. a. macrurus and R. a. hainanus was estimated to have occurred around 0.7–0.9 Ma (Figure 3D), coinciding with the period between the Xixiabangma glaciation (XG, 1.17–0.80 Ma) and Naynayxungla glaciation (NG, 0.78–0.50 Ma). After a brief population decline during the penultimate glaciation (PG), R. a. himalayanus underwent a rapid population expansion (Figure 3C). The divergence time between R. a. macrurus and R. a. hainanus occurred approximately 0.3 Ma (Figure 3D), coinciding with the emergence of the land bridge between Hainan Island and the mainland (0.3–0.13 Ma) during the Pleistocene, suggesting that this land bridge may have facilitated population migration and the subsequent formation of the insular endemic subspecies R. a. hainanus. Notably, R. a. hainanus exhibited a distinct population history marked by a sharp decline following its divergence from mainland populations. In addition to the lower genomic heterozygosity, reduced nucleotide diversity (Figure 3E), and slower linkage disequilibrium (LD) decay rate (Figure 3F), these results suggest that a genetic bottleneck occurred in this insular endemic subspecies after migration and subsequent isolation due to the disappearance of the land bridge.
A positive selection pipeline was applied to scan for significant genetic variations among R. affinis populations (details in Materials and Methods). This analysis identified 707 highly diverged genes in the high call frequency taxon (R. a. himalayanus) and 197 in the low call frequency taxa (R. a. macrurus and R. a. hainanus) (Figure 4A; Supplementary Tables S19, S20). Functional enrichment analysis of these genes showed a significant association with GO terms related to the renewal of cochlear hair cells and/or repair of hair cell damage, including regulation of mitotic cell cycle process, DNA damage response and DNA repair, and regulation of actin-based cytoskeleton organization (Supplementary Tables S21, S22).
To explore potential genotypic variations linked to the phenotypic differences in call frequencies, the identified PSGs were compared with known hearing-related genes associated with hearing loss and/or deafness in humans or mice (297 listed hearing loss/deafness-related genes from https://hereditaryhearingloss.org/ and He et al. (2021), Supplementary Table S23). The comparison identified nine highly diverged genes (GLI3, PCDH15, TECTA, WNT3A, EPS8L2, MPZL2, BIRC5, ACTG1, and NLRP3) functionally associated with hearing loss or deafness. Within these genes, GLI3 exhibited the highest FST and π values (Figure 4B). Genotyping results suggested two distinct genotypes between R. a. himalayanus and the other two subspecies (Figure 4B). The GLI3 gene, previously suggested to play a critical role in determining otocyst ventral polarity during inner ear development via the Sonic hedgehog (SHH) pathway (Ohta et al., 2016), contained 14 exons and was located on chromosome 17 in R. affinis. In this gene region, a total of 3 030 SNPs were identified, with 1 102 SNPs fixed in either R. a. himalayanus or the R. a. macrurus/R. a. hainanus group. Among these fixed SNPs, four were synonymous and one was nonsynonymous (Figure 4C; Supplementary Table S24). The nonsynonymous SNP results in an amino acid substitution from glutamate to lysine at the 776th amino acid site. Protein structure and functional predictions suggested that this substitution may severely disrupt the protein’s three-dimensional structure, likely leading to significant functional changes (Figure 4D). The other eight hearing-related genes identified under selection in R. affinis populations also showed functional associations with hair cell damage (PCDH15, Wagner & Shin, 2019; TECTA, Hildebrand et al., 2011; EPS8L2, Furness et al., 2013), protection of the inner ear against stress-induced cell damage (BIRC5, Habtemichael et al., 2010; WNT3A, Cui et al., 2023), actin-based hair cell cytoskeleton (ACTG1, Morín et al., 2009), structural integrity of the organ of Corti (MPZL2, Wesdorp et al., 2018), and reactive oxygen species (ROS)-, noise-, and aging-related hearing loss (NLRP3, Sai et al., 2022).
High-quality reference genomes play a crucial role in both comparative and population genomics. In this study, we constructed a chromosome-level genome assembly for R. affinis and performed comparative genomic analyses with seven other high-quality bat genomes.
Cytogenetic research on the Rhinolophus genus has previously suggested an ancestral karyotype of 2n=58, a pattern that closely resembles the current karyotype of R. ferrumequinum (Mao et al., 2007). Consistent with this, our whole-genome alignments across various bat species and other mammals supported the ancestral chromosomal structure of R. ferrumequinum chromosome 14 (Figure 1D). In addition, our findings supported chromosomal fissions and fusions as the main drivers of karyotypic evolution in Rhinolophus, analogous to the evolutionary processes observed in Erebia butterflies (Augustijnen et al., 2024). However, to fully elucidate the molecular mechanisms underlying chromosomal evolution in Rhinolophus, future studies will require chromosome-level genome assemblies from additional horseshoe bat species with lower chromosome numbers (e.g. 2n=28, 32, and 36).
Bats are well-established hosts of a wide array of viruses, including coronaviruses, rhabdoviruses, paramyxoviruses, and filoviruses (Tian et al., 2022). These viruses have the capacity to undergo cross-species transmission, posing significant pathogenic risks to other mammals, including humans, through direct contact or via intermediate hosts (Cui et al., 2019; Tian et al., 2022). Comparative genomic studies have provided insights into the genomic basis of virus tolerance in the ancestral Chiroptera lineage (Jebb et al., 2020; Moreno Santillán et al., 2021; Scheben et al., 2023; Zhang et al., 2013), revealing unique immune system adaptations in bats, such as loss of the PHYIN gene family (Ahn et al., 2016; Zhang et al., 2013), expansion and contraction of type I interferon (IFN) cytokines (Pavlovich, 2018; Zhou et al., 2016), and expansion of Tetherin (Hayward et al., 2022), PKR (Jacquet et al., 2022), and TNFRSF14 (Schneor et al., 2023). Given that a significant proportion of identified viruses (~80%) are hosted by species within the Vespertilionidae, Rhinolophidae and Pteropodidae families, research focusing on these specific clades may provide novel insights into the evolution and adaptive divergence of virus tolerance (Tian et al., 2022, 2023).
Comparative genomic analyses revealed that gene families expanded in Rhinolophidae, as well as PSGs, were enriched in immune-related functional categories, consistent with previous studies (Jebb et al., 2020; Moreno Santillán et al., 2021; Scheben et al., 2023; Zhang et al., 2013). Notably, we identified an expansion of MHC-II genes and HLA-DQB2 in Rhinolophidae, distinguishing them from other bats and mammals. While MHC-1 gene expansions have been documented in various bats, including those from Pteropodidae (Pavlovich, 2018), Noctilionidae, Mormoopidae, and Phyllostomidae (Moreno Santillán et al., 2021), the specific expansion of MHC-II genes and HLA-DQB2 in Rhinolophidae suggests a unique evolutionary adaptation to increased viral exposure. MHC-II molecules play a crucial role in initiating antigen-specific immune responses (Holling et al., 2004), and their expansion in Rhinolophidae may be associated with their role as primary hosts for coronaviruses, especially SARS-related coronaviruses (Ruiz-Aravena et al., 2022; Yan et al., 2021). Consistent with this, multiple PSGs in the Rhinolophidae clade were found to be involved in the SARS-CoV-2 signaling pathway, emphasizing their potential role in antiviral defense and providing support for the hypothesis that horseshoe bats may be a natural reservoir for SARS-CoV and SARS-CoV-2 (Ge et al., 2013; Temmam et al., 2022).
The high-quality chromosome-level reference genome of R. affinis enabled a comprehensive genome-wide scan for selection signals across the three R. affinis subspecies, presenting stable divergence in echolocation call frequencies. Consistent with previous studies (Mao et al., 2010, 2013; Mao & Rossiter, 2020), our analysis of whole-genome data from 21 individuals confirmed a closer relationship between R. a. macrurus and R. a. hainanus compared to R. a. himalayanus (Figure 3). These subspecies, which diverged relatively recently (0.7–0.9 Ma, Figure 3) and display distinct echolocation call frequencies, present a promising model for identifying candidate loci associated with this phenotypic variation. Positive selection analysis indicated that genes under positive selection in R. a. himalayanus populations, which utilize the highest echolocation call frequency, were primarily associated with adaptations to echolocation signal variation, including processes related to the renewal of cochlear hair cells (i.e., mitotic cell cycle process), repair of hair cell damage, and energy production. This finding aligns with the environmental differences between R. a. himalayanus and the other two subspecies, with the former relying on more intense echolocation calls and inhabiting noisier environments (Jakobsen et al., 2013). These results support the hypothesis that echolocation call divergence in bats is primarily driven by differential selection pressures in response to environmental adaptations (Jones & Holderied, 2007). Furthermore, given the importance of echolocation pulses in communication (Jones & Siemers, 2011), divergence in call frequency within R. affinis may lead to assortative mating, ultimately contributing to reproductive isolation and speciation, consistent with previous studies in horseshoe bats (Kingston & Rossiter, 2004). Acoustic divergence as a driver of speciation has been documented in numerous animals, including insects, frogs, birds, and mammals (reviewed in Wilkins et al., 2013). The multiple PSGs identified in this study, including nine known hearing/deafness-related genes, can be regarded as candidate ‘speciation genes’ (Nosil & Schluter, 2011) that encode a ‘magic trait’ (echolocation) involved in mating cues (Servedio et al., 2011). However, further studies incorporating whole-genome sequencing of additional individuals, as well as other methods, such as genome-wide association analysis, will be required to validate our current results.
In conclusion, this research has expanded our understanding of the genetic characteristics of horseshoe bats. Future studies with more high-quality genomic data from the Rhinolophidae family will be critical for delving deeper into the genetics of these bats and exploring potential links between their genetic traits and ecological or behavioral adaptations.
We would like to thank the National Engineering Laboratory for Resource Developing of Endangered Chinese Crude Drugs in Northwest China, Shaanxi Normal University. We are grateful to four anonymous reviewers whose comments improved the manuscript greatly.
The raw FASTQ sequences (BioProjectID PRJNA993631) and the reference genome assembly (accession number: JAUKPG000000000) of R. affinis were deposited in the NCBI database. The newly sequenced data were also submitted to GSA (BioProject: PRJCA026695) and Science Data Bank (CSTR: 31253.11.sciencedb.09440; DOI: 10.57760/sciencedb.09440). Codes used for comparative genomic and population analyses are available in the GitHub repository (https://github.com/GanglabSnnu/rafproj).
Supplementary data to this article can be found online.
The authors declare that they have no competing interests.
G.L. and X.M. designed the research; X.M. and W. C. provided the samples; L.Z. and J.Y. performed the genome assembly and annotation; J.Y., G.W., C.H., L.X., X.X., H.J., and T.S. analyzed the data; G.L., X.M., J.Y., G.W., L.Z., and L.X. wrote the original paper. All authors read and approved the final version of the manuscript.
Aggarwal G, Ramaswamy R. 2002. Ab initio gene identification: prokaryote genome annotation with GeneScan and GLIMMER. Journal of Biosciences, 27(1): 7–14. DOI: 10.1007/BF02703679
|
Ahn M, Cui J, Irving AT, et al. 2016. Unique loss of the PYHIN gene family in bats amongst mammals: Implications for inflammasome sensing. Scientific Reports, 6: 21722. DOI: 10.1038/srep21722
|
Augustijnen H, Bätscher L, Cesanek M, et al. 2024. A macroevolutionary role for chromosomal fusion and fission in Erebia butterflies. Science Advances, 10(16): eadl0989. DOI: 10.1126/sciadv.adl0989
|
Barcellos LF, May SL, Ramsay PP, et al. 2009. High-density SNP screening of the major histocompatibility complex in systemic lupus erythematosus demonstrates strong evidence for independent susceptibility regions. PLoS Genetics, 5(10): e1000696. DOI: 10.1371/journal.pgen.1000696
|
Bartek J, Bartkova J, Lukas J. 2007. DNA damage signalling guards against activated oncogenes and tumour progression. Oncogene, 26(56): 7773–7779. DOI: 10.1038/sj.onc.1210881
|
Belton JM, McCord RP, Gibcus JH, et al. 2012. Hi–C: a comprehensive technique to capture the conformation of genomes. Methods, 58(3): 268–276. DOI: 10.1016/j.ymeth.2012.05.001
|
Benedict CA, Norris PS, Ware CF. 2002. To kill or be killed: viral evasion of apoptosis. Nature Immunology, 3(11): 1013–1018. DOI: 10.1038/ni1102-1013
|
Cantalapiedra CP, Hernández-Plaza A, Letunic I, et al. 2021. eggNOG-mapper v2: functional annotation, orthology assignments, and domain prediction at the metagenomic scale. Molecular Biology and Evolution, 38(12): 5825–5829. DOI: 10.1093/molbev/msab293
|
Capella-Gutiérrez S, Silla-Martínez JM, Gabaldón T. 2009. trimAl: a tool for automated alignment trimming in large-scale phylogenetic analyses. Bioinformatics, 25(15): 1972–1973. DOI: 10.1093/bioinformatics/btp348
|
Chang SW, Fann CSJ, Su WH, et al. 2014. A genome-wide association study on chronic HBV infection and its clinical progression in male Han-Taiwanese. PLoS One, 9(6): e99724. DOI: 10.1371/journal.pone.0099724
|
Chen SF, Zhou YQ, Chen YR, et al. 2018. fastp: an ultra-fast all-in-one FASTQ preprocessor. Bioinformatics, 34(17): i884–i890. DOI: 10.1093/bioinformatics/bty560
|
Csorba G, Hutson A, Rossiter S, et al. 2019. Family rhinolophidae (horseshoe bats). In: Wilson DE, Mittermeier RA. Handbook of the Mammals of the World: vol. 9: Bats. Barcelona: Lynx Edicions, 260–332.
|
Cui FY, Cao ZM, Zhang QR, et al. 2023. The protective role of Wnt3a in peroxynitrite-induced damage of cochlear hair cells in vitro. Brazilian Journal of Otorhinolaryngology, 89(4): 101278. DOI: 10.1016/j.bjorl.2023.101278
|
Cui J, Li F, Shi ZL. 2019. Origin and evolution of pathogenic coronaviruses. Nature Reviews Microbiology, 17(3): 181–192. DOI: 10.1038/s41579-018-0118-9
|
Danecek P, Auton A, Abecasis G, et al. 2011. The variant call format and VCFtools. Bioinformatics, 27(15): 2156–2158. DOI: 10.1093/bioinformatics/btr330
|
De Bie T, Cristianini N, Demuth JP, et al. 2006. CAFE: a computational tool for the study of gene family evolution. Bioinformatics, 22(10): 1269–1271. DOI: 10.1093/bioinformatics/btl097
|
Ding YT, Chen WL, Li QQ, et al. 2021. Mitonuclear mismatch alters nuclear gene expression in naturally introgressed Rhinolophus bats. Frontiers in Zoology, 18(1): 42. DOI: 10.1186/s12983-021-00424-x
|
Dobin A, Davis CA, Schlesinger F, et al. 2013. STAR: ultrafast universal RNA-seq aligner. Bioinformatics, 29(1): 15–21. DOI: 10.1093/bioinformatics/bts635
|
Dudchenko O, Batra SS, Omer AD, et al. 2017. De novo assembly of the Aedes aegypti genome using Hi-C yields chromosome-length scaffolds. Science, 356(6333): 92–95. DOI: 10.1126/science.aal3327
|
Durand NC, Robinson JT, Shamim MS, et al. 2016a. Juicebox provides a visualization system for Hi-C contact maps with unlimited zoom. Cell Systems, 3(1): 99–101. DOI: 10.1016/j.cels.2015.07.012
|
Durand NC, Shamim MS, Machol I, et al. 2016b. Juicer provides a one-click system for analyzing loop-resolution Hi-C experiments. Cell Systems, 3(1): 95–98. DOI: 10.1016/j.cels.2016.07.002
|
Emms DM, Kelly S. 2015. OrthoFinder: solving fundamental biases in whole genome comparisons dramatically improves orthogroup inference accuracy. Genome Biology, 16(1): 157. DOI: 10.1186/s13059-015-0721-2
|
Feng S, Wan W, Li Y, et al. 2023. Transcriptome‐based analyses of adaptive divergence between two closely related spruce species on the Qinghai–Tibet Plateau and adjacent regions. Molecular Ecology, 32(2): 476–491. DOI: 10.1111/mec.16758
|
Foley NM, Springer MS, Teeling EC. 2016. Mammal madness: is the mammal tree of life not yet resolved?. Philosophical Transactions of the Royal Society B: Biological Sciences, 371(1699): 20150140. DOI: 10.1098/rstb.2015.0140
|
Funk WC, Lovich RE, Hohenlohe PA, et al. 2016. Adaptive divergence despite strong genetic drift: genomic analysis of the evolutionary mechanisms causing genetic differentiation in the island fox (Urocyon littoralis). Molecular Ecology, 25(10): 2176–2194. DOI: 10.1111/mec.13605
|
Furness DN, Johnson SL, Manor U, et al. 2013. Progressive hearing loss and gradual deterioration of sensory hair bundles in the ears of mice lacking the actin-binding protein Eps8L2. Proceedings of the National Academy of Sciences of the United States of America, 110(34): 13898–13903.
|
Ge XY, Li JL, Yang XL, et al. 2013. Isolation and characterization of a bat SARS-like coronavirus that uses the ACE2 receptor. Nature, 503(7477): 535–538. DOI: 10.1038/nature12711
|
Grabherr MG, Haas BJ, Yassour M, et al. 2011. Full-length transcriptome assembly from RNA-Seq data without a reference genome. Nature Biotechnology, 29(7): 644–652. DOI: 10.1038/nbt.1883
|
Guan DF, McCarthy SA, Wood J, et al. 2020. Identifying and removing haplotypic duplication in primary genome assemblies. Bioinformatics, 36(9): 2896–2898. DOI: 10.1093/bioinformatics/btaa025
|
Haas BJ, Papanicolaou A, Yassour M, et al. 2013. De novo transcript sequence reconstruction from RNA-seq using the Trinity platform for reference generation and analysis. Nature Protocols, 8(8): 1494–1512. DOI: 10.1038/nprot.2013.084
|
Haas BJ, Salzberg SL, Zhu W, et al. 2008. Automated eukaryotic gene structure annotation using EVidenceModeler and the Program to Assemble Spliced Alignments. Genome Biology, 9(1): R7. DOI: 10.1186/gb-2008-9-1-r7
|
Habtemichael N, Heinrich UR, Knauer SK, et al. 2010. Expression analysis suggests a potential cytoprotective role of Birc5 in the inner ear. Molecular and Cellular Neuroscience, 45(3): 297–305. DOI: 10.1016/j.mcn.2010.07.003
|
Hayward JA, Tachedjian M, Johnson A, et al. 2022. Unique evolution of antiviral tetherin in bats. Journal of Virology, 96(20): e01152–22.
|
He ZH, Ding YY, Mu YR, et al. 2021. Stem cell-based therapies in hearing loss. Frontiers in Cell and Developmental Biology, 9: 730042. DOI: 10.3389/fcell.2021.730042
|
Hildebrand MS, Morín M, Meyer NC, et al. 2011. DFNA8/12 caused by TECTA mutations is the most identified subtype of nonsyndromic autosomal dominant hearing loss. Human Mutation, 32(7): 825–834. DOI: 10.1002/humu.21512
|
Holling TM, Schooten E, van Den Elsen PJ. 2004. Function and regulation of MHC class II molecules in T-lymphocytes: of mice and men. Human Immunology, 65(4): 282–290. DOI: 10.1016/j.humimm.2004.01.005
|
Hu J, Fan JP, Sun ZY, et al. 2020. NextPolish: a fast and efficient genome polishing tool for long-read assembly. Bioinformatics, 36(7): 2253–2255. DOI: 10.1093/bioinformatics/btz891
|
Hughes JF, Coffin JM. 2001. Evidence for genomic rearrangements mediated by human endogenous retroviruses during primate evolution. Nature Genetics, 29(4): 487–489. DOI: 10.1038/ng775
|
Jacquet S, Culbertson M, Zhang C, et al. 2022. Adaptive duplication and genetic diversification of protein kinase R contribute to the specificity of bat-virus interactions. Science Advances, 8(47): eadd7540. DOI: 10.1126/sciadv.add7540
|
Jakobsen L, Brinkløv S, Surlykke A. 2013. Intensity and directionality of bat echolocation signals. Frontiers in Physiology, 4: 89.
|
Jebb D, Huang ZX, Pippel M, et al. 2020. Six reference-quality genomes reveal evolution of bat adaptations. Nature, 583(7817): 578–584. DOI: 10.1038/s41586-020-2486-3
|
Jones G, Holderied MW. 2007. Bat echolocation calls: adaptation and convergent evolution. Proceedings of the Royal Society B: Biological Sciences, 274(1612): 905–912. DOI: 10.1098/rspb.2006.0200
|
Jones G, Siemers BM. 2011. The communicative potential of bat echolocation pulses. Journal of Comparative Physiology A, 197(5): 447–457. DOI: 10.1007/s00359-010-0565-x
|
Jones G, Teeling EC. 2006. The evolution of echolocation in bats. Trends in Ecology & Evolution, 21(3): 149–156.
|
Keilwagen J, Wenk M, Erickson JL, et al. 2016. Using intron position conservation for homology-based gene prediction. Nucleic Acids Research, 44(9): e89. DOI: 10.1093/nar/gkw092
|
Kingston T, Rossiter SJ. 2004. Harmonic-hopping in Wallacea's bats. Nature, 429(6992): 654–657. DOI: 10.1038/nature02487
|
Kochi Y, Yamada R, Kobayashi K, et al. 2004. Analysis of single‐nucleotide polymorphisms in Japanese rheumatoid arthritis patients shows additional susceptibility markers besides the classic shared epitope susceptibility sequences. Arthritis & Rheumatism, 50(1): 63–71.
|
Kolmogorov M, Yuan J, Lin Y, et al. 2019. Assembly of long, error-prone reads using repeat graphs. Nature Biotechnology, 37(5): 540–546. DOI: 10.1038/s41587-019-0072-8
|
Kumar S, Suleski M, Craig JM, et al. 2022. TimeTree 5: an expanded resource for species divergence times. Molecular Biology and Evolution, 39(8): msac174. DOI: 10.1093/molbev/msac174
|
Kurtz S, Phillippy A, Delcher AL, et al. 2004. Versatile and open software for comparing large genomes. Genome Biology, 5(2): R12. DOI: 10.1186/gb-2004-5-2-r12
|
Lees-Miller SP, Meek K. 2003. Repair of DNA double strand breaks by non-homologous end joining. Biochimie, 85(11): 1161–1173. DOI: 10.1016/j.biochi.2003.10.011
|
Lenormand C, Bausinger H, Gross F, et al. 2012. HLA-DQA2 and HLA-DQB2 genes are specifically expressed in human Langerhans cells and encode a new HLA class II molecule. The Journal of Immunology, 188(8): 3903–3911. DOI: 10.4049/jimmunol.1103048
|
Li G, Wang JH, Rossiter SJ, et al. 2008. The hearing gene Prestin reunites echolocating bats. Proceedings of the National Academy of Sciences of the United States of America, 105(37): 13959–13964.
|
Li H, Durbin R. 2010. Fast and accurate long-read alignment with Burrows–Wheeler transform. Bioinformatics, 26(5): 589–595. DOI: 10.1093/bioinformatics/btp698
|
Li H, Durbin R. 2011. Inference of human population history from individual whole-genome sequences. Nature, 475(7357): 493–496. DOI: 10.1038/nature10231
|
Li H, Handsaker B, Wysoker A, et al. 2009. The sequence alignment/map format and SAMtools. Bioinformatics, 25(16): 2078–2079. DOI: 10.1093/bioinformatics/btp352
|
Liu Y, Han NJ, Franchini LF, et al. 2012. The voltage-gated potassium channel subfamily KQT member 4 (KCNQ4) displays parallel evolution in echolocating bats. Molecular Biology and Evolution, 29(5): 1441–1450. DOI: 10.1093/molbev/msr310
|
Majoros WH, Pertea M, Salzberg SL. 2004. TigrScan and GlimmerHMM: two open source ab initio eukaryotic gene-finders. Bioinformatics, 20(16): 2878–2879. DOI: 10.1093/bioinformatics/bth315
|
Mao XG, He GM, Hua PY, et al. 2013. Historical introgression and the persistence of ghost alleles in the intermediate horseshoe bat (Rhinolophus affinis). Molecular Ecology, 22(4): 1035–1050. DOI: 10.1111/mec.12154
|
Mao XG, Nie WH, Wang JH, et al. 2007. Karyotype evolution in Rhinolophus bats (Rhinolophidae, Chiroptera) illuminated by cross-species chromosome painting and G-banding comparison. Chromosome Research, 15(7): 835–848. DOI: 10.1007/s10577-007-1167-5
|
Mao XG, Rossiter SJ. 2020. Genome-wide data reveal discordant mitonuclear introgression in the intermediate horseshoe bat (Rhinolophus affinis). Molecular Phylogenetics and Evolution, 150: 106886. DOI: 10.1016/j.ympev.2020.106886
|
Mao XG, Zhu GJ, Zhang SY, et al. 2010. Pleistocene climatic cycling drives intra‐specific diversification in the intermediate horseshoe bat (Rhinolophus affinis) in Southern China. Molecular Ecology, 19(13): 2754–2769. DOI: 10.1111/j.1365-294X.2010.04704.x
|
Marçais G, Kingsford C. 2011. A fast, lock-free approach for efficient parallel counting of occurrences of k-mers. Bioinformatics, 27(6): 764–770. DOI: 10.1093/bioinformatics/btr011
|
McKenna A, Hanna M, Banks E, et al. 2010. The Genome Analysis Toolkit: a MapReduce framework for analyzing next-generation DNA sequencing data. Genome Research, 20(9): 1297–1303. DOI: 10.1101/gr.107524.110
|
Moreno Santillán DD, Lama TM, Gutierrez Guerrero YT, et al. 2021. Large‐scale genome sampling reveals unique immunity and metabolic adaptations in bats. Molecular Ecology, 30(23): 6449–6467. DOI: 10.1111/mec.16027
|
Morín M, Bryan KE, Mayo-Merino F, et al. 2009. In vivo and in vitro effects of two novel gamma-actin (ACTG1) mutations that cause DFNA20/26 hearing impairment. Human Molecular Genetics, 18(16): 3075–3089. DOI: 10.1093/hmg/ddp249
|
Nguyen LT, Schmidt HA, von Haeseler A, et al. 2015. IQ-TREE: a fast and effective stochastic algorithm for estimating maximum-likelihood phylogenies. Molecular Biology and Evolution, 32(1): 268–274. DOI: 10.1093/molbev/msu300
|
Nosil P, Schluter D. 2011. The genes underlying the process of speciation. Trends in Ecology & Evolution, 26(4): 160–167.
|
Ohta S, Wang B, Mansour SL, et al. 2016. SHH ventralizes the otocyst by maintaining basal PKA activity and regulating GLI3 signaling. Developmental Biology, 420(1): 100–109. DOI: 10.1016/j.ydbio.2016.10.004
|
Patterson N, Price AL, Reich D. 2006. Population structure and eigenanalysis. PLoS Genetics, 2(12): e190. DOI: 10.1371/journal.pgen.0020190
|
Pavlovich SS. 2018. Genomic Analysis and Examination of Innate Antiviral Immunity in the Egyptian Rousette Bat. Ph. D. dissertation, Boston University, Boston.
|
Purcell S, Neale B, Todd-Brown K, et al. 2007. PLINK: a tool set for whole-genome association and population-based linkage analyses. The American Journal of Human Genetics, 81(3): 559–575. DOI: 10.1086/519795
|
Ranallo-Benavidez TR, Jaron KS, Schatz MC. 2020. GenomeScope 2.0 and Smudgeplot for reference-free profiling of polyploid genomes. Nature Communications, 11(1): 1432. DOI: 10.1038/s41467-020-14998-3
|
Ruiz-Aravena M, McKee C, Gamble A, et al. 2022. Ecology, evolution and spillover of coronaviruses from bats. Nature Reviews Microbiology, 20(5): 299–314. DOI: 10.1038/s41579-021-00652-2
|
Sai N, Yang YY, Ma L, et al. 2022. Involvement of NLRP3-inflammasome pathway in noise-induced hearing loss. Neural Regeneration Research, 17(12): 2750. DOI: 10.4103/1673-5374.339499
|
Sanderson MJ. 2003. r8s: inferring absolute rates of molecular evolution and divergence times in the absence of a molecular clock. Bioinformatics, 19(2): 301–302. DOI: 10.1093/bioinformatics/19.2.301
|
Scheben A, Mendivil Ramos O, Kramer M, et al. 2023. Long-read sequencing reveals rapid evolution of immunity-and cancer-related genes in bats. Genome Biology and Evolution, 15(9): evad148. DOI: 10.1093/gbe/evad148
|
Schneor L, Kaltenbach S, Friedman S, et al. 2023. Comparison of antiviral responses in two bat species reveals conserved and divergent innate immune pathways. iScience, 26(8): 107435. DOI: 10.1016/j.isci.2023.107435
|
Schnitzler HU, Moss CF, Denzinger A. 2003. From spatial orientation to food acquisition in echolocating bats. Trends in Ecology & Evolution, 18(8): 386–394.
|
Servedio MR, Van Doorn GS, Kopp M, et al. 2011. Magic traits in speciation: ‘magic’ but not rare?. Trends in Ecology & Evolution, 26(8): 389–397.
|
Shao Y, Zhou L, Li F, et al. 2023. Phylogenomic analyses provide insights into primate evolution. Science, 380(6648): 913–924. DOI: 10.1126/science.abn6919
|
Simmons NB, Cirranello AL. 2024[2024-02-15]. Bat Species of the World: a taxonomic and geographic database. Version 1.5.
|
Sotero-Caio CG, Baker RJ, Volleth M. 2017. Chromosomal evolution in chiroptera. Genes, 8(10): 272. DOI: 10.3390/genes8100272
|
Stamatakis A. 2014. RAxML version 8: a tool for phylogenetic analysis and post-analysis of large phylogenies. Bioinformatics, 30(9): 1312–1313. DOI: 10.1093/bioinformatics/btu033
|
Stanke M, Keller O, Gunduz I, et al. 2006. AUGUSTUS: ab initio prediction of alternative transcripts. Nucleic Acids Research, 34(S2): W435–W439.
|
Stoffberg S, Jacobs DS, Mackie IJ, et al. 2010. Molecular phylogenetics and historical biogeography of Rhinolophus bats. Molecular Phylogenetics and Evolution, 54(1): 1–9. DOI: 10.1016/j.ympev.2009.09.021
|
Sun KP, Luo L, Kimball RT, et al. 2013. Geographic variation in the acoustic traits of greater horseshoe bats: testing the importance of drift and ecological selection in evolutionary processes. PLoS One, 8(8): e70368. DOI: 10.1371/journal.pone.0070368
|
Tamura K, Stecher G, Kumar S. 2021. MEGA11: molecular evolutionary genetics analysis version 11. Molecular Biology and Evolution, 38(7): 3022–3027. DOI: 10.1093/molbev/msab120
|
Teeling EC, Jones G, Rossiter SJ. 2016. Phylogeny, genes, and hearing: implications for the evolution of echolocation in bats. In: Fenton MB, Grinnell AD, Popper AN, et al. Bat Bioacoustics. New York: Springer, p25–54.
|
Temmam S, Vongphayloth K, Baquero E, et al. 2022. Bat coronaviruses related to SARS-CoV-2 and infectious for human cells. Nature, 604(7905): 330–336. DOI: 10.1038/s41586-022-04532-4
|
Tian J, Sun JM, Li DY, et al. 2022. Emerging viruses: cross-species transmission of coronaviruses, filoviruses, henipaviruses, and rotaviruses from bats. Cell Reports, 39(11): 110969. DOI: 10.1016/j.celrep.2022.110969
|
Tian SL, Zeng JM, Jiao HW, et al. 2023. Comparative analyses of bat genomes identify distinct evolution of immunity in Old World fruit bats. Science Advances, 9(18): eadd0141. DOI: 10.1126/sciadv.add0141
|
Traven A, Heierhorst J. 2005. SQ/TQ cluster domains: concentrated ATM/ATR kinase phosphorylation site regions in DNA-damage-response proteins. Bioessays, 27(4): 397–407. DOI: 10.1002/bies.20204
|
Wagner EL, Shin JB. 2019. Mechanisms of hair cell damage and repair. Trends in Neurosciences, 42(6): 414–424. DOI: 10.1016/j.tins.2019.03.006
|
Wang XY, Shi XL, Li Z, et al. 2006. Statistical inference of chromosomal homology based on gene colinearity and applications to Arabidopsis and rice. BMC Bioinformatics, 7: 447. DOI: 10.1186/1471-2105-7-447
|
Weckselblatt B, Rudd MK. 2015. Human structural variation: mechanisms of chromosome rearrangements. Trends in Genetics, 31(10): 587–599. DOI: 10.1016/j.tig.2015.05.010
|
Wesdorp M, Murillo-Cuesta S, Peters T, et al. 2018. MPZL2, encoding the epithelial junctional protein myelin protein zero-like 2, is essential for hearing in man and mouse. The American Journal of Human Genetics, 103(1): 74–88. DOI: 10.1016/j.ajhg.2018.05.011
|
Wilkins MR, Seddon N, Safran RJ. 2013. Evolutionary divergence in acoustic signals: causes and consequences. Trends in Ecology & Evolution, 28(3): 156–166.
|
Xie LF, Sun KP, Jiang TL, et al. 2017. The effects of cultural drift on geographic variation in echolocation calls of the Chinese rufous horseshoe bat (Rhinolophus sinicus). Ethology, 123(8): 532–541. DOI: 10.1111/eth.12627
|
Xu T, Sun MQ, Wang HT. 2017. Relationship between HLA-DQ gene polymorphism and hepatitis B virus infection. BioMed Research International, 2017: 9679843.
|
Yan H, Jiao HW, Liu QY, et al. 2021. ACE2 receptor usage reveals variation in susceptibility to SARS-CoV and SARS-CoV-2 infection among bat species. Nature Ecology & Evolution, 5(5): 600–608.
|
Yang ZH. 2007. PAML 4: phylogenetic analysis by maximum likelihood. Molecular Biology and Evolution, 24(8): 1586–1591. DOI: 10.1093/molbev/msm088
|
Yoshida K, Rödelsperger C, Röseler W, et al. 2023. Chromosome fusions repatterned recombination rate and facilitated reproductive isolation during Pristionchus nematode speciation. Nature Ecology & Evolution, 7(3): 424–439.
|
Zhang C, Dong SS, Xu JY, et al. 2019. PopLDdecay: a fast and effective tool for linkage disequilibrium decay analysis based on variant call format files. Bioinformatics, 35(10): 1786–1788. DOI: 10.1093/bioinformatics/bty875
|
Zhang GJ, Cowled C, Shi ZL, et al. 2013. Comparative analysis of bat genomes provides insight into the evolution of flight and immunity. Science, 339(6118): 456–460. DOI: 10.1126/science.1230835
|
Zhang JP, Zhang F, Tay WT, et al. 2022. Population genomics provides insights into lineage divergence and local adaptation within the cotton bollworm. Molecular Ecology Resources, 22(5): 1875–1891. DOI: 10.1111/1755-0998.13581
|
Zhang LB, Jones G, Zhang JS, et al. 2009. Recent surveys of bats (Mammalia: Chiroptera) from China. I. Rhinolophidae and Hipposideridae. Acta Chiropterologica, 11(1): 71–88. DOI: 10.3161/150811009X465703
|
Zhao HS, Sun S, Ding YL, et al. 2021. Analysis of 427 genomes reveals moso bamboo population structure and genetic basis of property traits. Nature Communications, 12(1): 5466. DOI: 10.1038/s41467-021-25795-x
|
Zhou P, Tachedjian M, Wynne JW, et al. 2016. Contraction of the type I IFN locus and unusual constitutive expression of IFN-alpha in bats. Proceedings of the National Academy of Sciences of the United States of America, 113(10): 2696–2701.
|
Zhou P, Yang XL, Wang XG, et al. 2020. A pneumonia outbreak associated with a new coronavirus of probable bat origin. Nature, 579(7798): 270–273. DOI: 10.1038/s41586-020-2012-7
|
Zima J, Volleth M, Horáček I, et al. 1992. Comparative karyology of rhinolophid bats (Chiroptera, Rhinolophidae). In: Horáček I, Vohralík V. Prague Studies in Mammalogy. Praha: Charles University Press, 229–236.
|
Type | G1 (Contig level) | G2 (Contig level) | G3 (Contig level) | Hi-C (Chromosome level) |
Number of contigs/scaffolds | 1 166 | 1 166 | 616 | 32 |
Contig/scaffold N50 (bp) | 30 381 862 | 30 560 113 | 30 201 243 | 92 909 886 |
Contig/scaffold N90 (bp) | 4 454 582 | 4 480 356 | 4 876 479 | 38 872 647 |
Longest contig/scaffold (bp) | 81 976 908 | 82 468 273 | 82 468 273 | 107 778 528 |
Average contig/scaffold length (bp) | 1 771 639 | 1 782 631 | 3 287 950 | 63 275 039 |
Total genome length (bp) | 2 065 732 003 | 2 078 548 679 | 2 025 377 528 | 2 024 801 270* |
*: Unanchored contig base count is not included. |